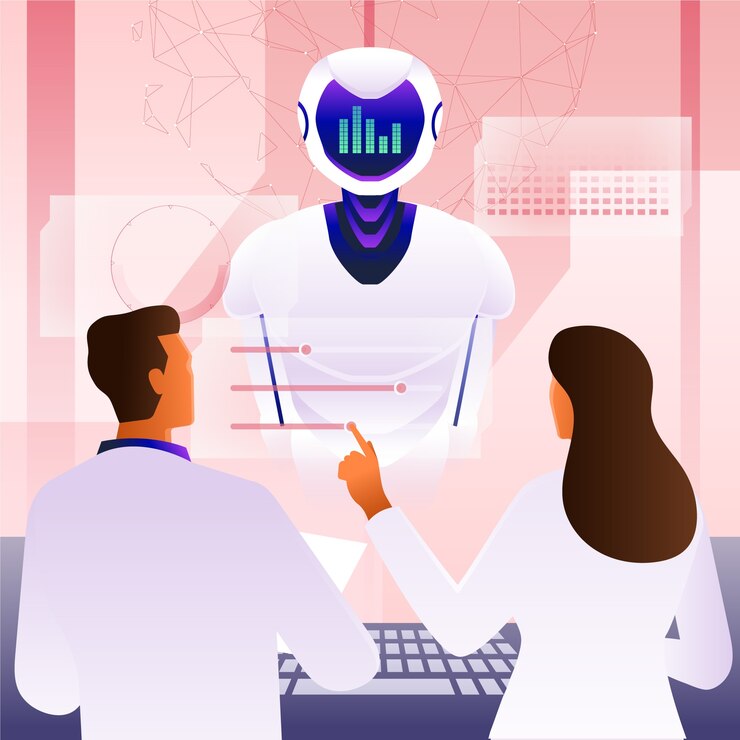
The Significance of Explainable AI
Consider the following scenario: an AI-driven system rejects a credit application. Without an explanation for the decision, the applicant is bewildered and frustrated. Such examples demonstrate the need of Explainable AI. By offering insights into the factors that influence decisions, XAI promotes trust and responsibility, both of which are critical components of AI adoption. Furthermore, in high-stakes fields such as healthcare and finance, where decisions affect people's lives and livelihoods, interpretability is not only desired, but necessary.
Understanding AI Decisions
At the heart of Explainable AI is the requirement to understand AI decisions. This includes breaking down the black box of complicated algorithms and explaining the reasoning behind their results. Techniques like as feature relevance analysis, model visualisation, and surrogate models help to clarify the relationship between input variables and predictions. Through intuitive visualisations and human-readable explanations, stakeholders obtain vital insights into AI-driven decisions, allowing them to verify, challenge, or revise them as needed.
Challenges to Achieving Explainable AI
Despite its importance, establishing Explainable AI presents numerous hurdles. The intrinsic complexity of AI models, together with the trade-off between performance and interpretability, is a significant challenge. Furthermore, the lack of standardised techniques and regulatory frameworks makes issues more complicated. Furthermore, maintaining interpretability across several domains and applications necessitates specialised techniques, which adds another degree of complexity to the XAI ecosystem.
The Function of Billing Software Development Services in Explainable AI
Explainable AI relies heavily on billing software development services. These services help firms properly evaluate and understand AI-driven decisions by utilising advanced analytics and machine learning approaches. Billing software developers make it easier to integrate XAI into existing workflows by creating unique solutions targeted to certain sectors and use cases, which improves transparency and accountability.
Custom CRM development in India is a catalyst for explainable AI adoption
In India, custom crm development india firms are leading the way in Explainable AI adoption. These companies build and deploy CRM solutions that incorporate XAI capabilities, leveraging their deep domain experience and technical acumen. Businesses can obtain a competitive advantage in the global economy by exploiting India's talented workforce and low-cost development resources. From predictive analytics to customer segmentation, Explainable AI-powered CRM platforms enable organisations to make educated decisions and provide personalised customer experiences.
Overcoming Challenges: Collaborative Solutions
Addressing the difficulties of Explainable AI necessitates collaborative solutions from stakeholders across sectors and disciplines. Multidisciplinary teams, including data scientists, domain specialists, ethicists, and policymakers, can collaborate to create standardised methodology and regulatory guidelines for XAI implementation. These combined efforts can accelerate Explainable AI adoption and realise its full potential across a wide range of applications by cultivating an ecosystem of knowledge exchange and cooperation.
Industry-specific applications
Explainable AI finds applications in a wide range of industries, each with its own set of obstacles and potential. In healthcare, XAI can improve clinical decision support systems, allowing doctors to interpret AI-generated diagnoses and treatment recommendations. Similarly, in finance, Explainable AI can help with risk assessment and fraud detection by offering transparency into the factors that influence lending choices and transactions. Businesses may exploit AI capabilities while remaining transparent and accountable by designing XAI solutions to suit sector requirements.
Ethical considerations
Ethical considerations are critical in the development and implementation of Explainable AI. As AI systems have more influence on society, concerns about bias, justice, and accountability emerge. Ethical AI frameworks, such as fairness-aware machine learning and algorithmic transparency, provide rules for reducing these dangers and ensuring that AI judgements follow ethical norms. Furthermore, continual communication and involvement with stakeholders, including as governments, civil society organisations, and the general public, is critical for negotiating the ethical challenges of Explainable AI.
Future Directions
Looking ahead, Explainable AI has the potential for continued innovation and evolution. Advances in model interpretability techniques, such as attention mechanisms and counterfactual explanations, are expected to improve the transparency and comprehensibility of AI systems even further. Furthermore, as the regulatory landscape changes, firms must adapt and adopt XAI as a fundamental principle of responsible AI implementation. Organisations may embrace the revolutionary potential of Explainable AI by staying up to date on emerging trends and best practices.
Addressing User Concerns and Empowering Individuals
Explainable AI benefits not only enterprises, but also individuals by giving them more knowledge and control over AI-driven decisions that touch their daily lives. Understanding why AI systems produce specific suggestions or forecasts gives consumers confidence and allows them to make more educated decisions. Explainable AI enables consumers to evaluate and trust AI-driven outcomes, whether they are making a personalised recommendation on an e-commerce platform or receiving a healthcare diagnosis, encouraging a more transparent and inclusive digital environment.
Education and Awareness Campaigns
To encourage widespread deployment of Explainable AI, education and awareness efforts are required. By demystifying difficult AI concepts and emphasising the significance of transparency and accountability, these projects encourage individuals and organisations to incorporate XAI principles into their daily operations. Raising awareness about the benefits and limitations of Explainable AI, through seminars, webinars, educational resources, and public forums, can spark important debates and create positive change in how AI technologies are perceived and implemented.
Global Collaboration and Knowledge Sharing
Explainable AI is a global initiative that crosses geographical boundaries and industry sectors. By encouraging worldwide collaboration and knowledge exchange, stakeholders can speed up the development and deployment of XAI technology. International venues, like as conferences and consortiums, offer excellent opportunities for researchers, practitioners, and policymakers to exchange ideas, discuss best practices, and tackle common obstacles in the quest of transparent and interpretable AI systems.
Democratising AI Access
In addition to transparency and interpretability, democratising access to AI technology is critical to unlocking Explainable AI's full potential. By making XAI tools and resources available to developers, entrepreneurs, and researchers around the world, we can democratise AI innovation and empower different communities to use AI for social benefit. Open-source efforts, collaborative platforms, and developer communities play critical roles in democratising AI access, allowing for greater participation and contributions to the XAI ecosystem.
Conclusion: A Vision for the Future
Finally, explaining Explainable AI is a cultural as well as technological need. By putting openness, accountability, and inclusion first in AI development and deployment, we may create a future in which AI-driven judgements are understandable, trustworthy, and consistent with human values. billing software development services and bespoke CRM development firms in India and throughout the world continue to advocate XAI ideals, paving the way for a more transparent and ethical AI landscape in which technology is used to drive positive change and empower all.